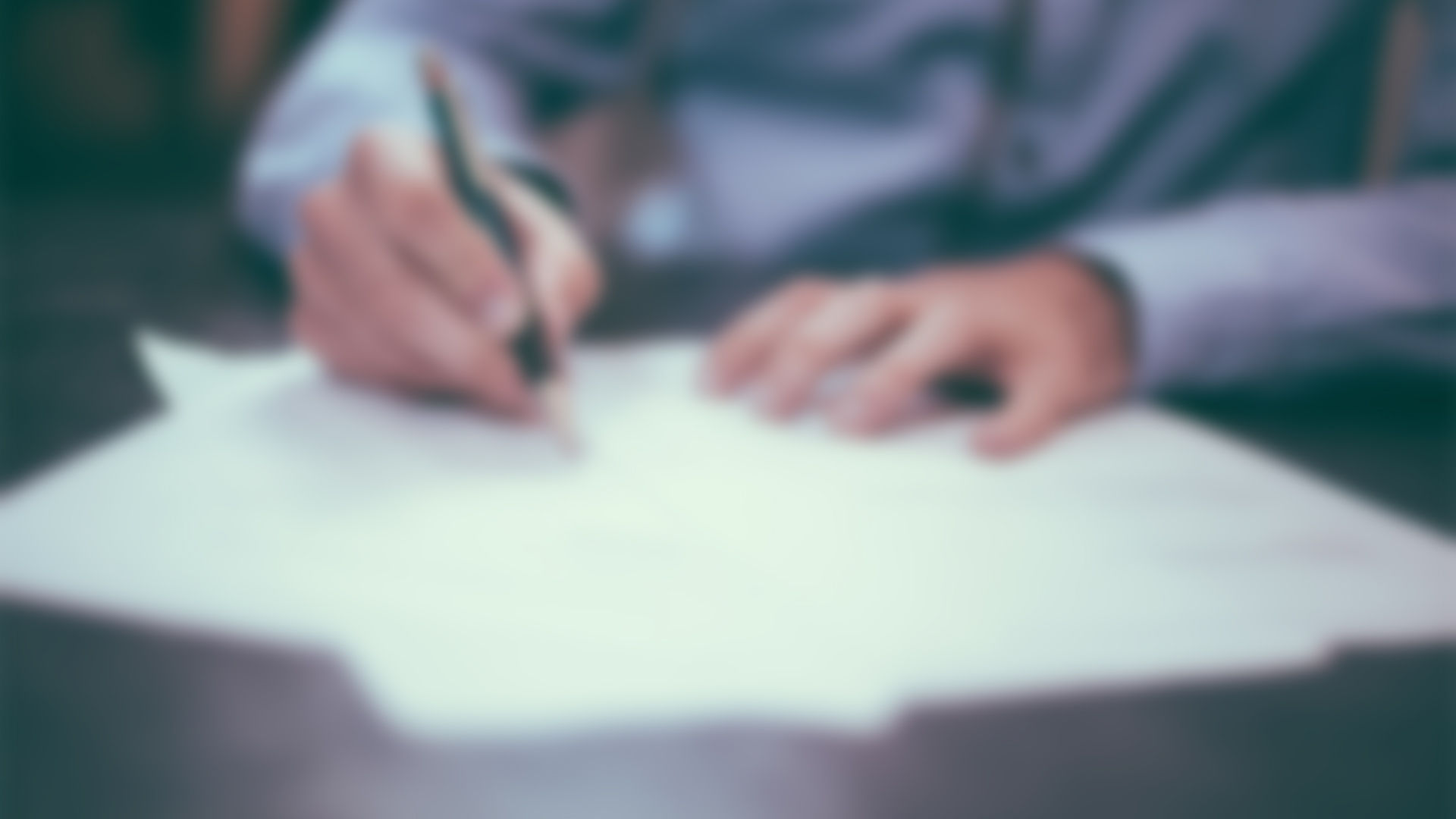
Ashley Paul Mundaden
Software Developer
Phase 1 Search
KnowYourCar
"See, when you drive home today, you've got a big windshield on the front of your car. And you've got a little bitty rear-view mirror. And the reason the windshield is so large and the rear-view mirror is so small is because what's happened in your past is not near as important as what's in your future."
One would think that the search criteria of a search box would be as simple as looking for the words in the database and get the relevant results back. Well, the rise of internet has lead to the rise of competition in all sectors. Keeping customers coming back to your website is an integral part of keeping your business relative in today's world. 'Search' plays a huge part in that as its the first frontier and what results we show the user will decide whether they want to stay on our portal or just hit the 'back' button. Let us delve deep into how I created my search engine for my app KnowYourCar
Explanation:
In this phase I have created a search box that gets your relevant rows from the data-set by calculating the Tf-IDF values of the search input and compare them with the TF-IDF values of each of the rows and return the one with highest cosine similarity.
The term frequency of a word in a document. There are several ways of calculating this frequency, with the simplest being a raw count of instances a word appears in a document.
The inverse document frequency of the word across a set of documents. This means, how common or rare a word is in the entire document set. The closer it is to 0, the more common a word is.This metric can be calculated by taking the total number of documents, dividing it by the number of documents that contain a word, and calculating the logarithm.
Every time we input a string we treat it as a document and perform TF-IDF calculation for the input doc. We create it in a vector form with the vector size being the size of number of words that were in the input and each value would be the corresponding TD-IDF score of the word. We then compare it with the TF-IDF calculation of the rows in the data-set for those words. When we compare we create a cosine similarity of our input vector with each of the rows and then sort to get the most relevant pages on top. These rows that were fetched will be more useful to the user compared to when doing a plain text search.
Contribution:
-
Created vectors for the query and calculated the TF-IDF to be later compared with the rows to fetch the most similar documents based on cosine similarity
-
Loaded all the data on server start up and maintained an inverted index.
-
Maintained the length of all the documents with their index
-
Sorted them based on similarity and sent them to UI.
Experiments:
Experiment 1
Affects of Different Stemmers
Tested for 1000 rows
PorterStemmer - 28077 words
Snowball Stemmer - 27941 words
Lancaster Stemmer - 27512 words (Winner)
Experiment 2
Affects of different Lemmatizers
Tested for 1000 rows
TextBlob Lemmatizer - 29557 words
WordNet Lemmatizer - 28995 words ( Winner)
Experiment 3
Affect of the Lemmatizer and Stemmer
Used WordNet Lemmatizer along with Lancaster Lemmatizer to see how many words could it reduce if used together
Original Number of words - 27512(LS)
After applying Lemmatizer - 27481 words
We happened to reduce 31 words which doesn't seem like a lot but it will have a large effect when we're dealing with billions of documents
Difficulties Faced:
-
Figuring out and creating an architecture for the project took a while
-
(Loading data in the start, creating an inverted index and calculating lengths based on index)
-
Grasping concepts of flask to render the data on the UI took a very good amount of time to execute
-
Calculating cosine similarity gave me a number of Nan values as well and it ruined my search results. Figuring it out and filtering it out of my results was an issue that took me a lot of debugging to understand
References:
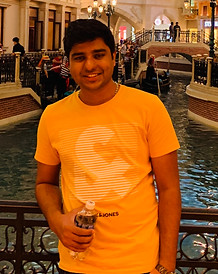