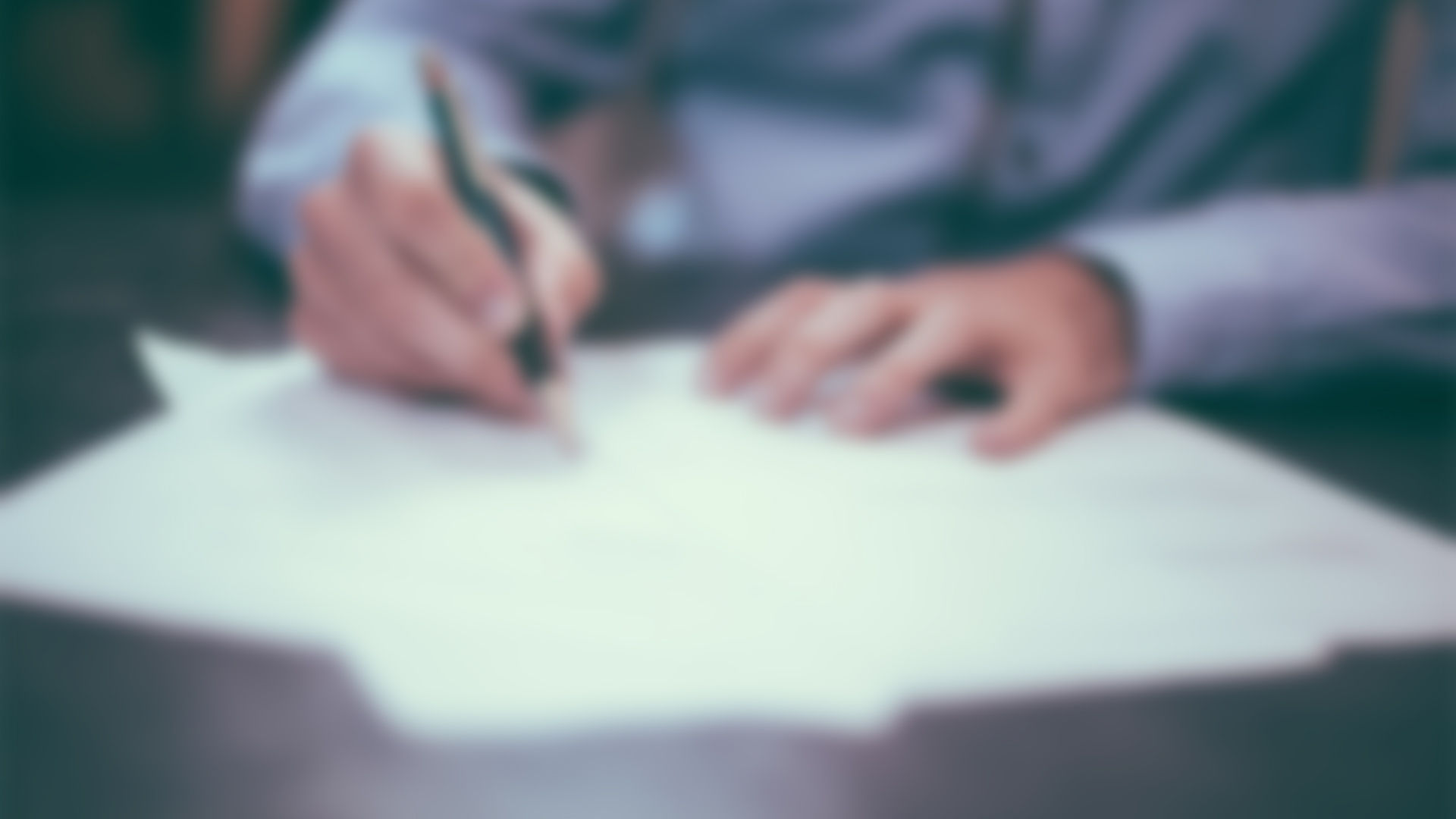
Ashley Paul Mundaden
Software Developer
Ashley Paul Mundaden
Phase 1 Search
Phase 2 Classifier
KnowYourCar
Things I learned:
-
Understanding of Naive-Bayes Theorem
-
Implementation of Naive Bayes Classification on a data-set
-
What is Hyper-parameter tuning and how it is implemented
-
What is a confusion matrix and how it helps us understand how good our classifier is
-
Overcoming problems listed at the end of the blog
Explanation:
The classifier works on a refurbished cars data-set from Kaggle . The data-set has a list of refurbished cars with a number of details and most importantly for this phase the owner's description about the car. The aim of this phase of my project is to analyze an input from the user and to classify it. After analyzing the input we predict what kind of car the text input matches to the most and return the results along with its probability back to the user.
​
We will be using the Naive Bayes theorem in order to classify data. The formula of Bayes theorem is as follows:
​
P(H|E) = (P(E|H) * P(H)) / P(E)
​
In this case we have more than one Hypothesis, so in order to find the Hypothesis with the highest probability we will need to calculate using this formula for all the Hypothesis.
​
So for phase 2 we first train the model, so when our server starts the first thing we do is read the data-set and train the model in order to use it later. In the training phase we calculate the number of labels that we have i.e. the number of Hypothesis that we need to test for.
P(H) = number of times the label has occurred / total rows
In order to calculate the P(E|H) we need to first maintain all the words that occurred in each label. For example
P(Sedan|awesome,car) =
(P(awesome|Sedan) * P(car|Sedan) * P(Sedan)) / P(awesome)*P(car)
​
Calculating this will give us the probability of these words belonging to the label Sedan. So to calculate this we need to find the probability of the words 'awesome' and 'car' in the label Sedan. So giving out another example to calculate the probability of awesome we need to do as follows:
P(awesome|Sedan) =
no. of rows with awesome strictly of label Sedan / no. of rows with label Sedan
​
Doing this will get you the evidence of each word and then you can implement it in the formula to get the final probability.
​
In the Classify phase we first take an input from the user, tokenize it to remove all the stop words as Stem and Lemmatize the words. The resultant words are then calculated for each of the hypothesis using all the lists and the maps that we maintained earlier in the training phase. In order to compare the probability of all the hypothesis we need to use Nai've Bayes Formula:
​
P(H|E) = (P(E|H) * P(H)) / ΣP(E|H)
​
Using this we will calculate the probability of each of the Hypothesis and we will have successfully classified the input.
Hyper-parameter Tuning:
In some cases there is a chance that we won't find a certain word from the input in our data-set. This mostly occurs when we misspell the words in the input. In such a case the probability of all the words i.e. P(E|H) will be 0, and since we multiply it with each other and if even one word's probability comes zero then the whole equation would come to be 0.
In order to tackle this issue we need to do Hyper-parameter Tuning also called as Smoothing. We add a dummy row to each of the evidence so even if any of the word is not there in the data-set it will always have value of 1 (it depends on what value you put for tuning). Below is a code snippet of me implementing this smoothing technique in my Naive-Bayes Classifier.
I experimented my classifier with various alpha values and the lower the alpha value that I put ,the better the accuracy i achieved
alpha value = 1 Accuracy = 30.2%
alpha value =0.001 Accuracy = 44.1%
alpha value =0.00001 Accuracy=45.4%
Confusion Matrix:
Below is the graph for the Confusion Matrix, Confusion matrix is a matrix that helps us understand the efficiency of the classifier. If the number of True-Positives and True Negatives are relatively higher it means that the Classifier is good and Vice versa.
Tackling issues:
With the low accuracy rate, I went on a quest to find out different ways that I can probably approach with which I can increase my accuracy measure. After reading the description of many cars that were listed I realized that there were a lot of rows with descriptions that would not help identify a specific car. There were descriptions that were basically advertisements of the car dealer with nothing written about the car itself. I realized that in my journey to get a better accuracy i definitely needed data that could help my classifier distinguish between different cars. After a lot of brainstorming and analyzing my data-set I came to a probable solution. I decided to train my classifier not just on the description but on other columns of the data-set as well that could help the classifier distinguish clearly between the different types of cars. So I added 5 more columns to of my data-set to the my classifier's training code, namely - price, manufacturer, condition, type and paint of the cars. So while training the data-set along with the descriptions I read the data from these columns and added these words in the dictionary. Feeling confident I trained the data set with 8000 rows and tested it with 2000 rows and much to my dismay the accuracy only increased by 2%. My new accuracy was now
47%. I wasn't ready to give up though, analyzing my data-set even more I realized that most of the test data(descriptions from the test data) also were not particularly talking about the car itself. Maybe that's the reason why I could not get a high accuracy. I decided that instead of training my data-set on the description as well as the columns, why don't I try to train it without the description column. Its just confusing the Classifier with data that won't help it achieve its objective. So, once again I trained the data and tested it. So when I didn't train the data-set with description I happened to get a lower accuracy then even before. So this basically means that not all my descriptions are bad. Many of them are pretty useful as well.
So i tried giving the data-set my own inputs and it is classifying them as per how I would like it. Its giving me correct types of cars based on what I'm putting into the input. I have written an Insight of my findings below that I hope would shed some more light on this issue.
Insight:
The reason for the low accuracy is because the model is trained on the description column. These descriptions are not necessarily describing the car, it varies from a person talking about how 'he wants to sell this car because his grandma is old and she can't drive it anymore' to as vague as 'I have a car". Yes surely with so many experiments on this data-set I found out that there were indeed a lot of descriptions in there with actually meaningful data. But then again it wasn't enough for a higher accuracy rate. Which is why the model cant predict correctly even after training it on 10,000 rows of data. If the descriptions was some official description written about the car then we would definitely see a higher accuracy in our model. Please do check the website as for user inputs it works fine and there is a very high probability that you will get your desired car. I tried every way to get the best results and I hope my Classifier blog will help you understand the ups & downs as well as the do's and don'ts that you need to keep in mind when you're making a Naive Bayes Classifier. There was a lot to learn in this phase and a lot concepts are clear to me after having worked on them practically and using them in my project.
Problems Faced:
-
Understanding the Naive Bayes Marginalization formula to calculate the probability of each Hypothesis when compared to each other
-
Running the experiments on my computer would take a lot of time and it would occasionally crash, used google colab to tackle this
-
Went through a learning curve to understand the Confusion Matrix
-
Understood the concept of Hyper-parameter tuning, used smoothing to avoid getting zero probabilities for misspelled words.
Contributions:
-
Wrote the whole code solely on the professor's input and understanding the concept of how the Classifier works( No in-built Libraries were used to calculate anything)
-
Created a logic to save values for generating a confusion matrix for all of my 13 types of cars.
-
Used Lancaster Stemmer as well as WordNet Lemmatizer to save similar words in turn saving space and time
-
Used different parameters for smoothing and recorded the Accuracy for each.
-
Created a confusion matrix for each of the Hypothesis to get a clear understanding of the efficiency of the classifier
References:
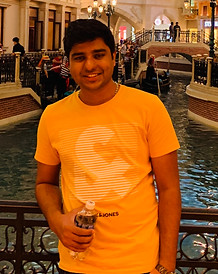
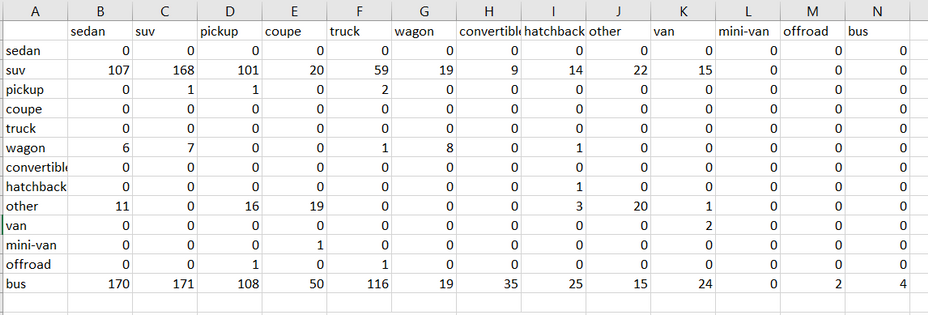