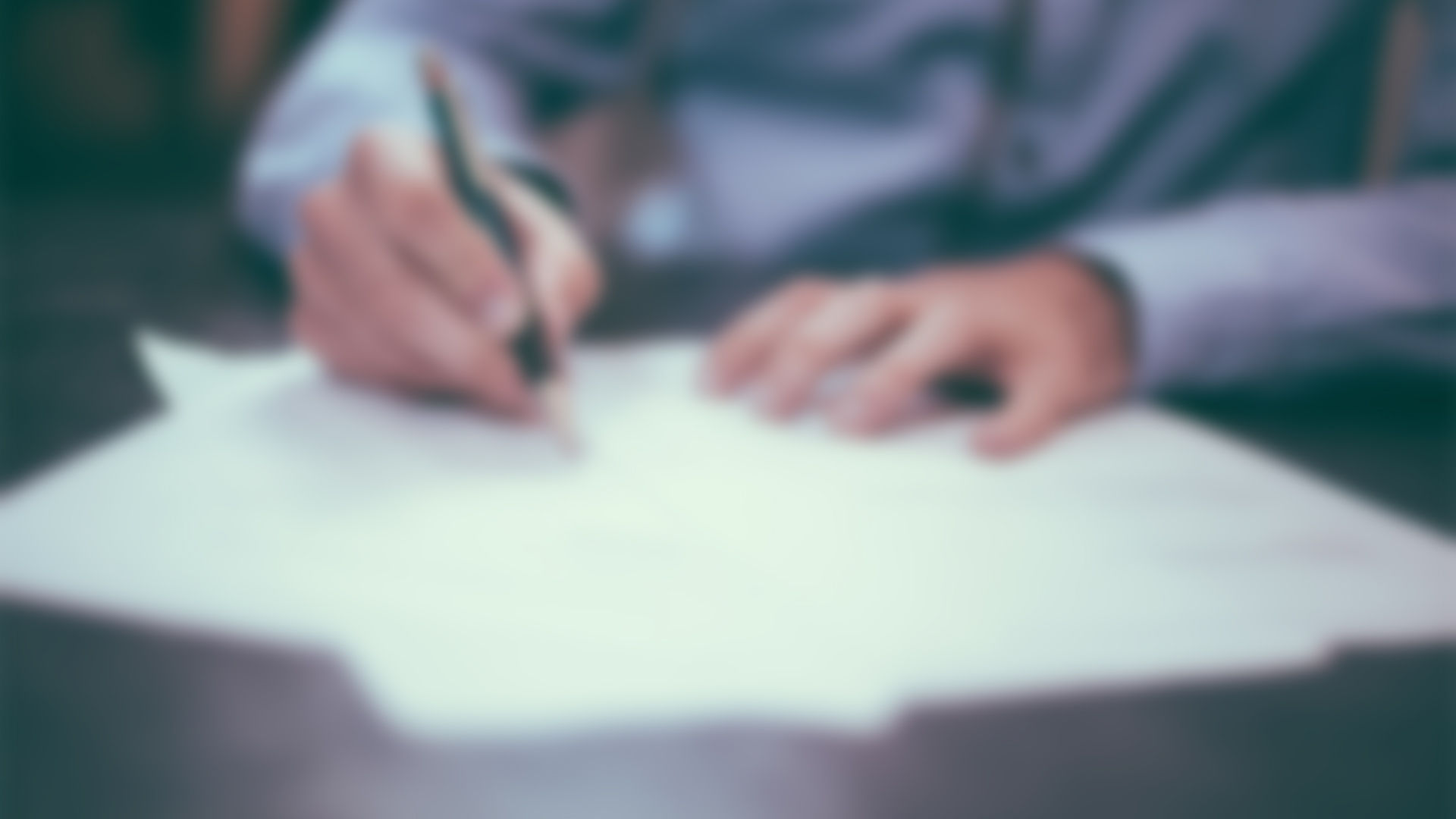
Ashley Paul Mundaden
Software Developer
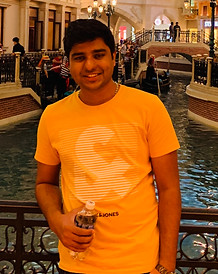
Phase 3 Image Captioning
KnowYourCar
Things I learned:
-
Understood the implementation of a Image captioning method using an attention-based model
-
Reverse engineering the attention based model to caption new images
-
Working with Jupyter Notebooks in Google Colab
Generating captions:
Image captioning is the process by which a computer assigns metadata in the form of captioning or keywords to a digital image. This application of computer vision techniques is used largely in image retrieval systems to organize and locate images of interest from a database. This concept of captioning an image was inconceivable in the past, but after the recent development in Deep Neural Networks or Deep learning it has become an easy problem given the correct data-set to train on.
​
As mentioned above in order to create a model that captions an image correctly we first need to train it on a suitable data-set. To do this we use the MS-COCO data-set. MS-COCO data-set has around 200k labelled images that is freely available for data science students and enthusiasts.
​
We first download the MS COCO data-set and then do some simple pre processing on it in order to prepare it.
​
Now we pre-process the images using TensorFlow's InceptionV3. InceptionV3 is used to classify each image by extracting features from the last convolutional layer. After all the images are passed we pickle all the data and save it to the disk.
​
We then tokenize all the captions which will give us all the vocabulary of all the unique words in the data. We will also pad all the sequences to be the same length as the longest one. After this we split the data into training set and testing set. We will use the training set in order to train the model and use the testing set to check the accuracy of this model.
​
We train the model by extracting the features stored in the respective .npy files and then pass those features through the encoder. The encoder output, hidden stat(initialized to 0) and the decoder input(which is the start token) is pass to the decoder.The decoder returns the predictions and the decoder hidden state.The decoder hidden state is then passed back into the model and the predictions are used to calculate the loss.Use teacher forcing to decide the next input to the decoder.Teacher forcing is the technique where the target word is passed as the next input to the decoder.The final step is to calculate the gradients and apply it to the optimizer and backpropagate.
Below is the graph for that shows the loss changes to the Epoch
​
​
​
​
​
​
​
​
​​​​​​​
After we train the model I wrote a code to read my UsedCars Data-set and to process all the images in it and generate captions for it. These captions are then saved and i store them in my dataset and then write it inorder to use it later for my image search
Image Search with text:
Now that I have created the data-set we need to implement it in order to Search for an image. As mentioned in the Search phase we read the csv file and then create an inverted index of all the words in the caption that occurred. I tokenize it using Lancaster Stemmer and Wordnet Lemmatizer and also remove all repeating words in order to create a unique set of words.
When an input text is received we first go create its input vector. I then compare this input vector with the vectors of each row and calculate their similarity using cosine similarity. I then rank the rows based on similarity and return the top results that were found. For a more in depth understanding of the TF-IDF search please read my Phase 1 Search blog.
Image Search with input image:
I would like to start off this column by stating that if you want to host your project on PythonAnywhere you will definitely have to buy a paid plan. I used TensorFlow 1.15.0 and this package alone is of 480 mb. You get around 500 mb on PythonAnywhere on a free account and that won't suffice.
​
There are a number of things you need to prep in order to implement image search in your project, Lets start with preping the data.
​
First, we need to train our model with the MS-COCO data-set that I mentioned above. Only this time we need to make sure that we train it by altering the method gru by making it return only CPU compatible layers. This ensures that the data that is created during training will be compatible with CPU and CPU supported websites.
​
Now after training the data-set for about 4 horus we need to save the values that were changed/modified during training and download them into our computer so we can test it with new images.
This is a list of files that you'll need to download after the training is complete:
​
After this we need to make sure that we have TensorFlow 1.xx installed in your IDE. On server start up you need to load all this data onto your class objects and keep them ready in order to process new images. We will then ask the user for an input image. We will first save the input image into a directory in our server and save the path. We will then then use this image path to send that url to the model so it can process the image and generate the captions. Once the captions are generate we take the caption and use it as an input to our Image Search with text method and get the relevant results.
Predicted Caption Examples:
Efforts:
I am mentioning my efforts because I learnt a lot in this phase about a lot of things and I would like to mention as many things as i can that I overcame working in this phase of the project.
​
I knew in the start that in order to create captions for new images I would definitely need to save the values that were created/modified during the training period of the MS-COCO dataset. So after the training I saved the weights and I proceeded to create the model in order to create the captions. I loaded the weights and instantiated the objects and gave an input image in order to test if a caption gets generated that i can make sense of. The caption of the first image was created "a man a man a man a man a man a man a man a man a man a man <end>"
My model seemed correct and I wasn't sure the reason for this caption so I uploaded an another image to get a caption " steeple steeple steeple steeple .." and so on. Not sure what was wrong I started comparing the dimensions of the image input on colab and on my local machine to find that there were quite a few differences. I started researching and reading about this issue and I happened to read this blog where it said that I needed to train my model such that it works on CPU powered machines as well(mentioned above the code change that I had to make). After doing that I acquired new weights that would hopefully generate better captions. I updated my Tensorflow package from 2.0.0 to 1.15.00 and ran the model again with the same caption and low and behold i got the caption " a car in the side of the road".
This whole process helped me grasp the concept of how image captioning works very quickly and how training on a GPU or CPU affects the behaviour of the model so distinctively.
In my attempt at hosting my "Search with image input" on python anywhere I faced an issue of not having and disk space left on the server. The free version of pythonanywhere lets you have 500 mb of free space and Tensorflow 1.15.0 is alone 480 mb. I have updated the code for this on github in the relevant class mentioned in the Readme file. I have also shown its functionality in the project video that you can find here.
Problems Faced:
-
Understanding the concepts of an Attention based model
-
Took time understanding the different behaviour of the model in GPU vs CPU run machines
-
Training the model on Google Colab took 4 hours which was very time consuming
-
While training the model it would occasionally disconnect which will result in running the whole training from scratch
-
As i was fairly new to working with Jupyter Notebook and Google colab it took me some time to understand how to work with it
Contributions:
-
Wrote code to create captions for new images by saving the values that were created or modified while training the model.
-
Wrote a code that inputs all the images from my dataset to the trained model on google colab and save the captions that got generated to the data-set
-
Used Lancaster Stemmer as well as WordNet Lemmatizer to save similar words in turn saving space and time
-
Reverse engineered the whole Tensor flow image captioning code to create captions for new images but it would create insensible captions(Currently working on fixing that issue)